Everything about business and society is changing due to the impact of technology and the deluge of data.
Data Science is an interdisciplinary activity that uses concepts from statistics, mathematics, software engineering and artificial intelligence to extract information from data in order to make better decisions and to understand and analyse real facts in detail.
When applied to organisations, Data Science helps to analyse, interpret and answer complex business questions through the interdisciplinary application of statistics, mathematics, data modelling and visualisation, big data and artificial intelligence (machine learning, deep learning). In other words, Data Science enables organisations to make strategic, tactical and operational decisions driven by data.

Data Science gives us answers to what exists, will exist and, is not obvious or known.
We are fully in the information and digitalisation era where the intensive and extensive use of data (variety, volume and velocity), statistics, mathematics, predictive and explanatory models and fact-based management are fundamental to support the decision-making process, the creation of competitive advantages and the generation of value in organisations and society. Situations are analysed with the data generated, in order to have control over what is happening in the present and predict what may happen in the future.
And so, with this, organisations are emerging that are 100% connected in real time (people, processes and things), interactive, integrated, flexible, mobile, intelligent, sustainable and social, i.e., Organisations 4.0.
Traditional reporting or dashboards, by their nature, are based on the idea of reacting to what happened in the past "reactive analytics" whereas today's more innovative and valuable trends integrate "prescriptive analytics", which tell us how to face the future.
Prescriptive analytics is more than just making predictions. Predictions play an important role, of course, but prescriptive analytics is about answering questions about what we should do, once we have our predictions.
Raw data without the right processing is of no use for conclusions or valuable information. That is why the correct organisation and visualisation of data (data mining & data visualisation) allow us to make sense of the data, to put all the pieces together to "tell" stories of high business value (Data Story).

Data is transformed into information and information into insights.
Social Labs, through its Social Business Platforms and its Data Science unit made up of a multidisciplinary team of data scientists, data analysts, data engineers, engineers and software and cloud computing specialists, offer a very differentiating value to its clients by converting a "deluge" of data into actionable business knowledge in real time:
1. Planning
Before looking for answers in the data, the needs and objectives of the business must be well defined. These will define the lines of research to be addressed analytically.
2. Data collection and storage
Through the Social Advisors by Social Labs platform, all kinds of consumer and customer data can be captured in real time (insights), executing numerous and varied missions, such as surveys, innovation and co-innovation, creation and co-creation, recording evidence of consumption and purchase, evidence of inventory, price elasticity, competitor analysis, market share, brand tracking, product evaluation, product presentation, planogram evaluation, communication test, usability test, digital behaviour and influence zone, mystery shopper, tracking & journey, focus groups and individual or group empathy conversations, etc.
3. Data cleaning and preparation
The data has to be processed and sorted so that it is consistent and useful for finding the answers you are looking for.
4. Data analysis
In this phase, an exploratory analysis of the data is carried out, and models are created and optimised. To do so, tools such as machine learning, deep learning and statistical models are usually used; R programming, Python, Julia, Google AI Machine Learning, Microsoft Azure Machine Learning Studio, Amazon SageMaker, DataRobot, RapidMiner, among others, are tools and platforms commonly used in Data Science.
5. Comprehensive visualisation
To get this point right, it is necessary to work on making the data easy to understand and extract knowledge from it.
6. Insights
With a good application of Data Science, we can obtain crucial information such as predicting behaviours, recommendation models by habits or tastes, fraud detection, establishing patterns and trends, which will lead to recommendations to boost a business.
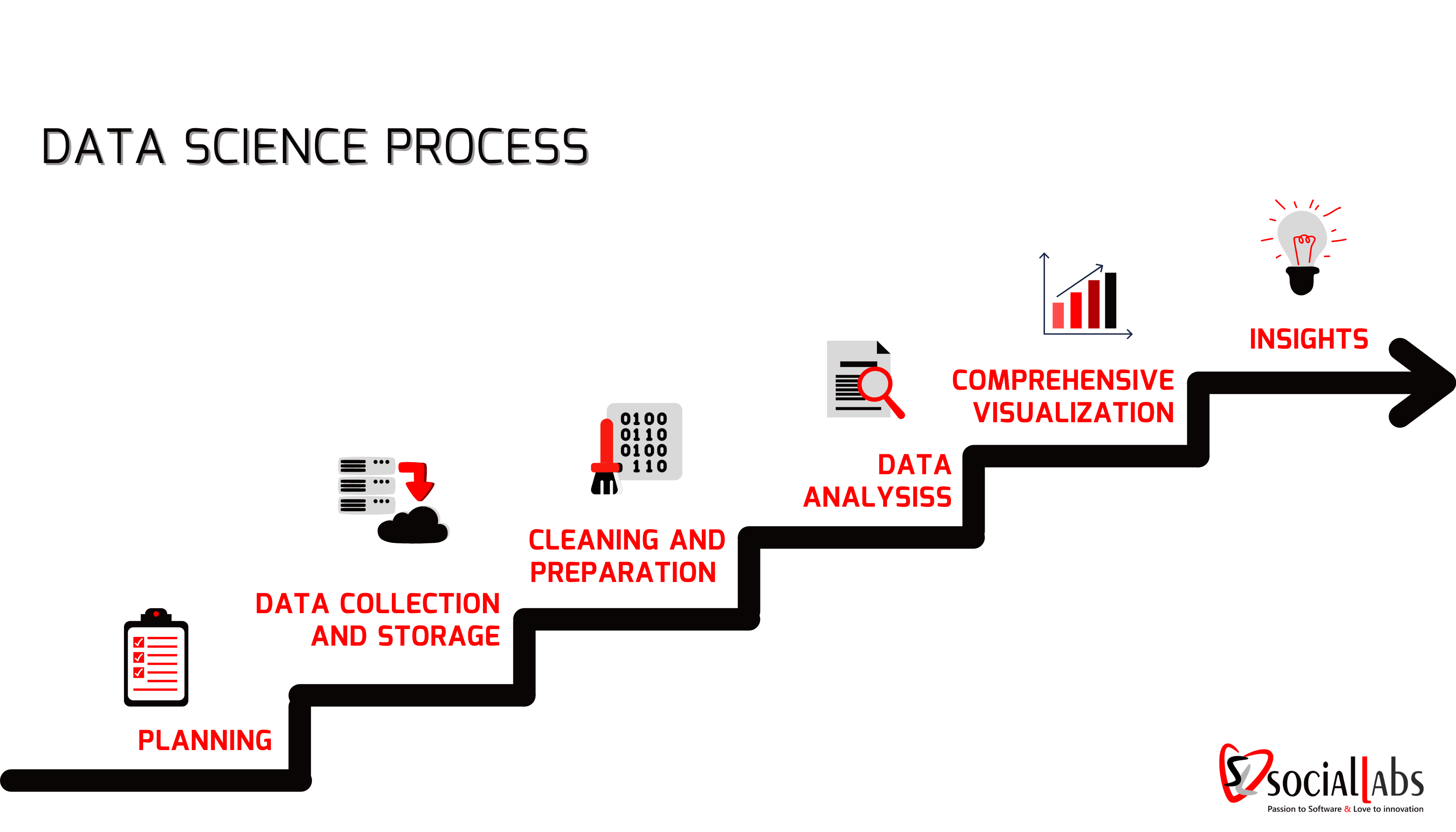
At Social Labs we apply this Data Science process to help our clients, such as Bimbo Group or Ab Inbev, to make the best "quasi-in-real-time" business decisions. Decisions such as what new product they should launch, demand projections, trend and behaviour forecasting, and with the business intelligence extracted from the data we advise them on how to maximise their profits, generate innovation and strategic business advantages.